Computer scientists achieve what was “impossible” a few years ago
Researchers can now use AI to extract vital information from a breast cancer biopsy in seconds rather than weeks.
They’re harnessing the power of deep learning to discover whether a particular protein is preventing the patient’s own body from attacking a tumor.
A team of computer scientists at the Technion – Israel Institute of Technology, in Haifa, has achieved what was thought to be impossible only a few years ago.
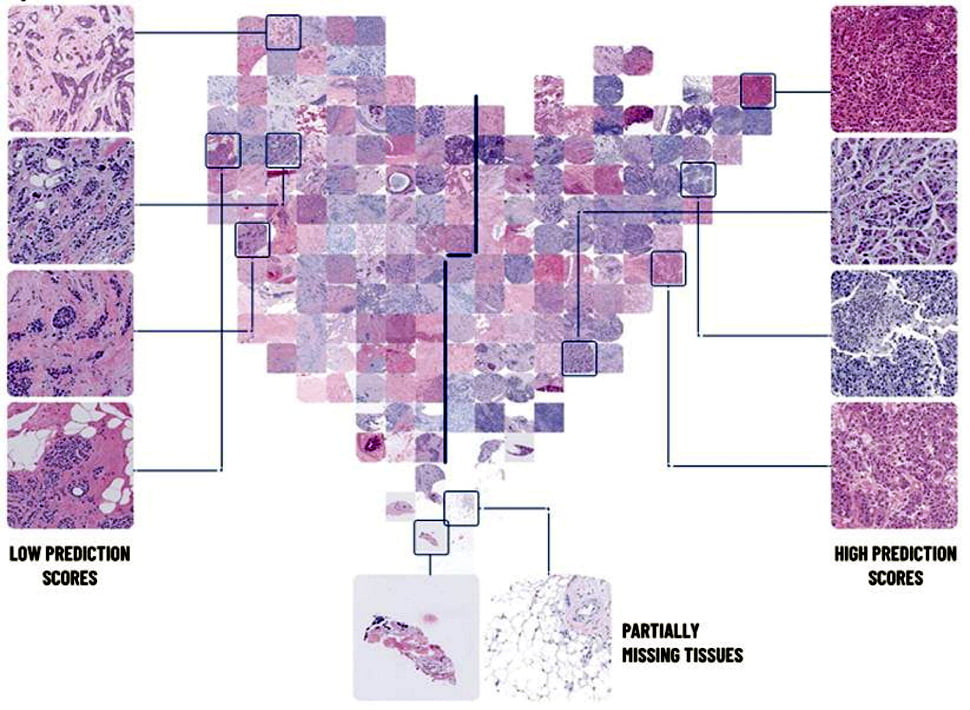
They have trained a computer to determine the presence of PD-L1, a protein that effectively provides “password protection” for the tumor, and stops the immune system from attacking it.
Armed with that knowledge, doctors can go on to prescribe immunotherapy drugs which are able to break the password and allows the body’s defenses to kick in.
The researchers taught the AI to identify PD-L1 by showing it biopsies from thousands of patients.
In tests the neural networks – or artificial ways of “thinking” – that they developed were able to correctly determine the presence of PD-L1 in 70 per cent of cases. In the other 30 per cent of cases the AI wasn’t wrong – it just wasn’t able to reach a conclusion.
It’s a huge step forward, that could ultimately lead to machines diagnosing patients, although it’s yet to reach the success rates of human pathologists.
Having said that, it is far quicker, far cheaper, and it has the potential to become more consistent and more reliable.
Doctors currently send a patient’s biopsy, or tissue sample, to the pathology lab. A basic test using two simple dyes – hematoxylin and eosin, (known as H&E staining) – is enough to tell whether the patient has cancer, and how advanced it is.
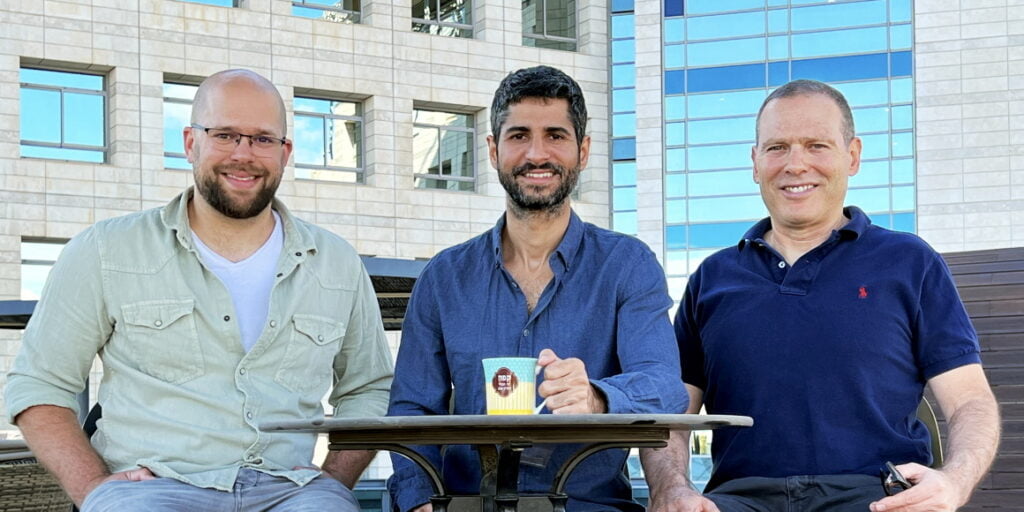
But further analysis of that biopsy, to establish the presence of PD-L1, requires much more intricate staining and is a long and complex procedure. A patient will typically wait two weeks or more for the lab to give them a yes or no.
Prof Ron Kimmel, Dr Gil Shamai and Amir Livne, all working at the Technion’s Taub Faculty of Computer Science, realized that AI may offer a solution.
They reasoned that it may be powerful enough to see patterns in the “basic test” that would allow it to determine the presence of PD-L1 without the advanced tests at the pathology lab.
They were right. They know it works because they’ve compared its findings with those of human pathologists. They know it’s looking for visual patterns no human could detect. But as is the way with so-called “black box AI”, they don’t know what they are.
“One question we’re always being asked, at least by doctors is how the hell AI managed to see that,” Dr Shamai tells NoCamels.
“We invented the AI, we built it, and we made it work, and we know that it’s working.
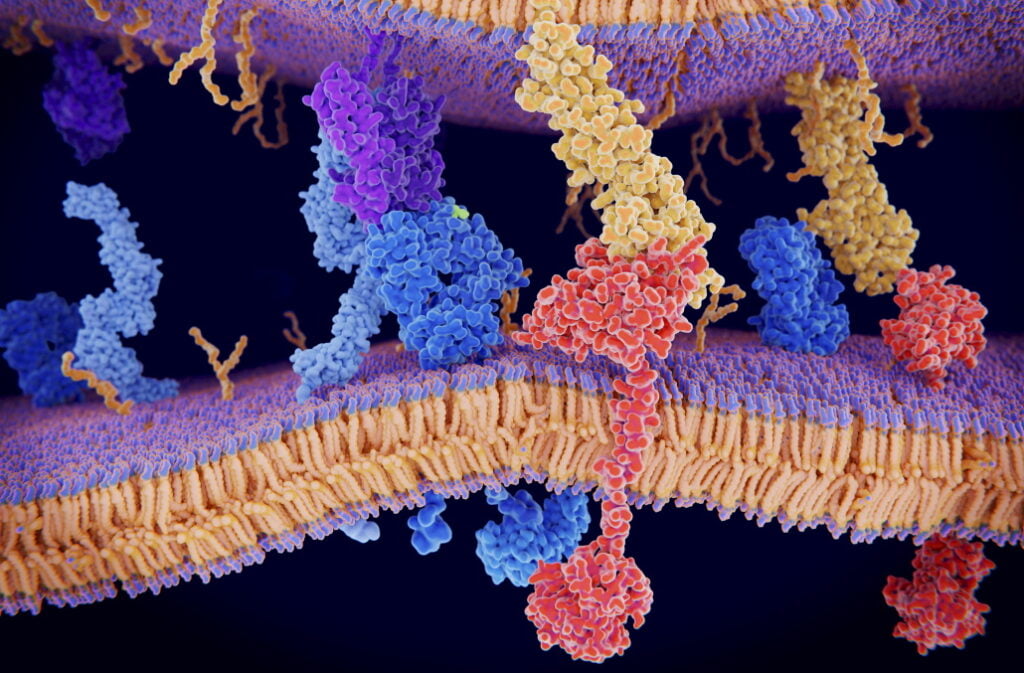
“We know the exact computation, but the computation is complex and we cannot comprehend it and really understand how the prediction was done.
“This is a general thing in advanced AI, it’s considered black box, it can do amazing things, but you can’t interpret them in an intuitive manner.”
A study detailing their research, entitled Deep learning-based image analysis predicts PD-L1 status from H&E-stained histopathology images in breast cancer, was recently published in Nature Communications.
Sign up for our free weekly newsletter
Subscribe“This is a momentous achievement,” said Prof Kimmel. “The variations that the computer found are not distinguishable to the human eye.
“Cells arrange themselves differently if they present PD-L1 or not, but the differences are so small that even a trained pathologist can’t confidently identify them. Now our neural network can.”
The AI looks at a digital scan of the patient’s biopsy and gives a near-instant answer.
The team trained it with digital biopsy images from 3,376 patients that were tagged as either expressing PD-L1, or not.
They then tested it with 275 images it hadn’t seen before, to assess whether it could correctly predict the presence of PD-L1. It was right 70 per cent of the time, and in 30 per cent of cases it was unable to say, because it couldn’t spot a pattern.
In the cases where it disagreed with the human pathologist, a second test proved the AI to be right.
“Seven years ago a clinician came up with this idea that maybe we can predict a lot of these proteins by applying artificial intelligence to a digital scan of the biopsy, with just the basic staining,” said Dr Shamai.
“It sounded like a crazy idea. They told us it couldn’t be done so of course, we had to prove them wrong.”
Scanning biopsies rather than viewing them through a microscope is a recent innovation, as are the advances in deep learning. Together they’re what made the team’s breakthrough possible.
But they still had to locate enough scans to teach the AI, and they faced numerous challenges because the files were enormous, and came in a variety of formats that had to be standardized.
“We’ve been growing these in the last three years. I have expanded this idea, with collaborations with medical centers and more data,” said Dr Shamai.
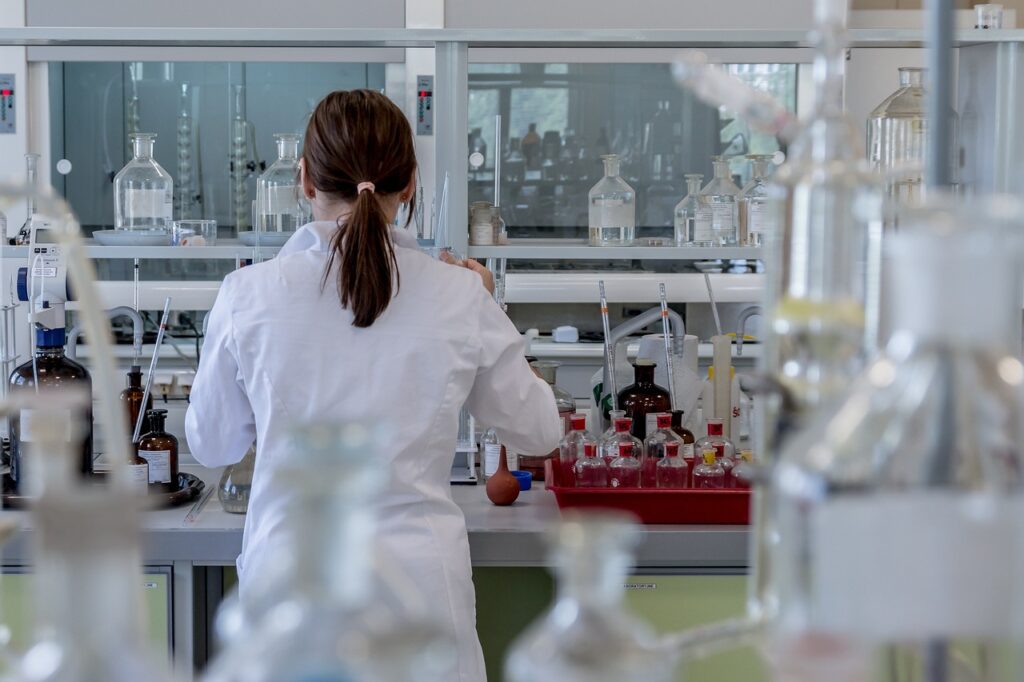
“We now have more clinicians, pathologists and oncologists advising us. And now we have a team of around 19 students, 12 doing their first degree, and more who are master’s or PhD students.
“We have three developers who are working with us, we have two students from the Faculty of Medicine doing the data collection and we have many more ongoing studies.”
At present the test can be used as quality assurance to check the work of human pathologists, but the team is refining the technology all the time. Dr Shamai believes that, in time, it could be authorized by the FDA for use as a diagnostic tool.
Prof Kimmel said: “We expect AI to become a powerful tool in doctors’ hands. AI can assist in making or verifying a diagnosis, it can help match the treatment to the individual patient, it can offer a prognosis.
“I do not think it can, or should, replace the human doctor. But it can make some elements of doctors’ work simpler, faster, and more precise.”
Related posts
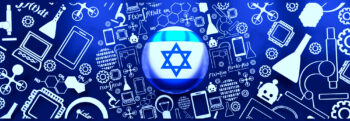
Israeli Medical Technologies That Could Change The World
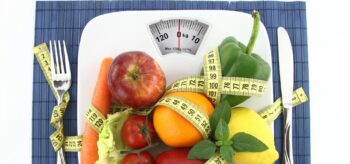
Harnessing Our Own Bodies For Side Effect-Free Weight Loss
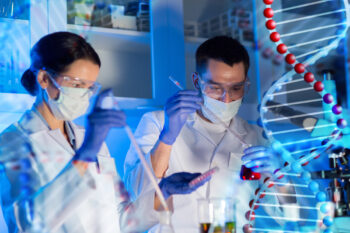
Missing Protein Could Unlock Treatment For Aggressive Lung Cancer
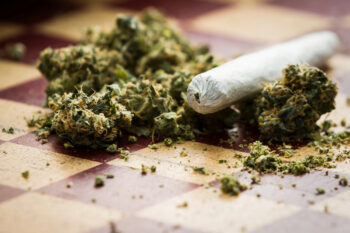
Facebook comments