With conspiracy theories and so-called fake news rampant on social media, in particular during major election periods, researchers at Ben-Gurion University of the Negev (BGU) have developed a method to help fact checkers keep up with increasing volumes of misinformation on these platforms.
A team led by Dr. Nir Grinberg and Prof. Rami Puzis of BGU’s Department of Software and Information Systems Engineering found that tracking fake news sources, rather than individual articles or posts, can significantly lower the burden on fact checkers and produce reliable results over time.
The researchers’ audience-based models outperformed the more common approach of looking at who’s sharing misinformation by large margins: 33 percent when looking at historical data, and 69 percent when looking at sources as they emerge over time.
The authors also showed that their approach can maintain the same level of accuracy in identifying fake news sources while requiring less than a quarter of the fact-checking costs.
“The problem today with the proliferation of fake news is that fact checkers are overwhelmed,” explained Grinberg.
“They cannot fact check everything … [and] we know little about how successful fact checkers are in getting to the most important content to fact check. That prompted us to develop a machine learning approach that can help fact checkers direct their attention better and boost their productivity,” he said.
The team’s findings were published recently in Proceedings of the 30th ACM SIGKDD Conference on Knowledge Discovery and Data Mining.
Related posts
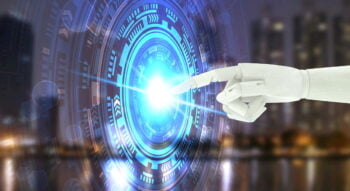
Israeli AI Safety Tool Among TIME’S Best Inventions For 2024
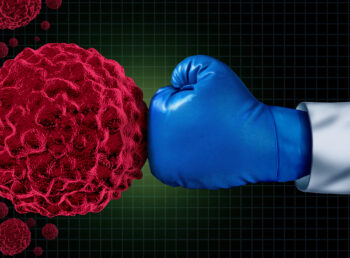
TAU Team Discovers Mechanism To Eliminate Cancerous Tumors
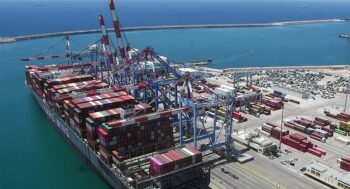
Ashdod Port Investing In Startups As Part Of Innovation Strategy
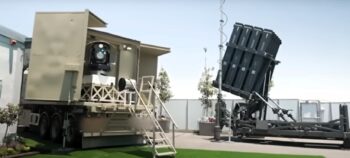
Facebook comments