Israel startup Run:AI exited stealth mode today with the announcement of $13 million in funding for its virtualization and acceleration solution for deep learning. Run:AI bridges the gap between data science and computing infrastructure by creating a complete virtualization layer for deep learning, speeding up the training of neural network models. The funding included a $10 million Series A round led by Haim Sadger’s S Capital and TLV Partners, coming after a seed round of $3 million from TLV Partners.
Deep learning uses neural networks that mimic some functions of the human brain, and is the most complex and advanced type of AI, powering functions like image recognition, autonomous vehicles, smart assistants like Alexa and Siri, and much more.
Deep learning needs to be trained in order to work, a process that takes time and significant computing power. Companies often run deep learning workloads on a very large number of Graphical Processing Units (GPUs) or specialized AI cores. These workloads run continuously for days or weeks on expensive on-premises computers or cloud-based providers.
The time and cost of training new models are the biggest barriers to creating new AI solutions and bringing them quickly to market. Deep learning requires experimentation, and slightly-modified training workloads could be run hundreds of times before they’re accurate enough to use. This results in very long times-to-delivery, as workflow complexities and costs grow.
Run:AI says it has completely rebuilt the software stack for deep learning to get past the limits of traditional computing, claiming it makes training faster, cheaper and more efficient.
“Traditional computing uses virtualization to help many users or processes share one physical resource efficiently; virtualization tries to be generous,” said Omri Geller, Run:AI co-founder and CEO. “But a deep learning workload is essentially selfish since it requires the opposite: it needs the full computing power of multiple physical resources for a single workload, without holding anything back. Traditional computing software just can’t satisfy the resource requirements for deep learning workloads.
Rona Segev-Gal, Managing Partner of TLV Partners, said, “Executing deep neural network workloads across multiple machines is a constantly moving target, requiring recalculations for each model and iteration based on availability of resources. Run:AI determines the most efficient and cost-effective way to run a deep learning training workload, taking into account the network bandwidth, compute resources, cost, configurations and the data pipeline and size.” she said.
In stealth since it was founded in 2018, the company has already signed several early customers internationally and has established a US office.
Related posts
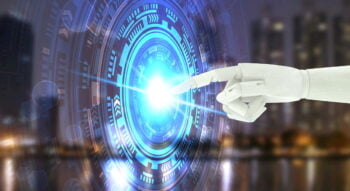
Israeli AI Safety Tool Among TIME’S Best Inventions For 2024
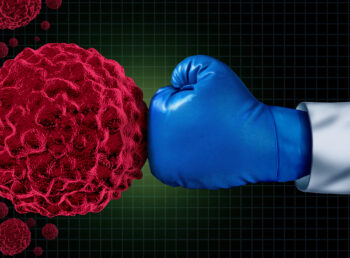
TAU Team Discovers Mechanism To Eliminate Cancerous Tumors
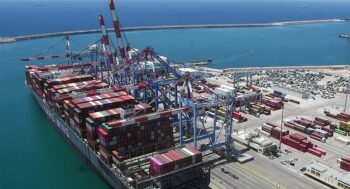
Ashdod Port Investing In Startups As Part Of Innovation Strategy
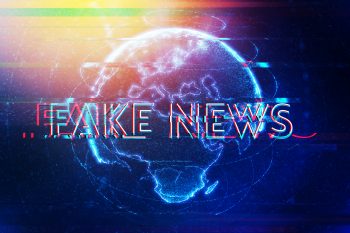
Facebook comments